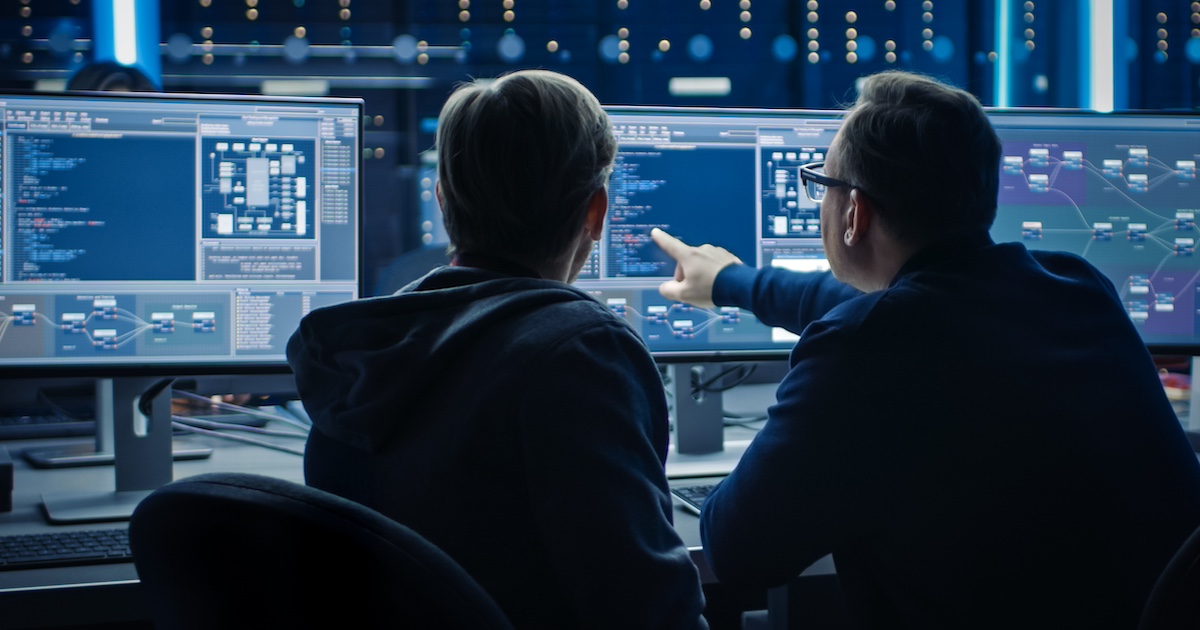
Apple researchers have released a study highlighting the limitations of large language models (LLMs), concluding that LLMs’ genuine logical reasoning is fragile and that there is “noticeable variance” in how models respond to different examples or representations of the same question.
The researchers analyzed the formal reasoning capabilities of LLMs, particularly in mathematics.
They noted that the GSM8K benchmark, widely used to assess the mathematical reasoning of models on grade-school-level questions, has significantly improved in recent years. Still, it remains unclear if the mathematical reasoning capabilities have advanced, citing questions of the reliability of the reported metrics.
Therefore, to evaluate the models, researchers conducted a large-scale study using numerous state-of-the-art open and closed models and introduced GSM-Symbolic, “an improved benchmark created from symbolic templates that allow for the generation of a diverse set of questions” aimed at overcoming limitations that exist in evaluations.
Researchers found fragility in the mathematical reasoning of the models and that its performance significantly declined as the number of clauses in a question increased.
The researchers hypothesized that the deterioration was caused by the fact that current LLMs are not capable of genuine logical reasoning, but instead, they tried to replicate the steps noted in their training data.
“When we add a single clause that appears relevant to the question, we observe significant performance drops (up to 65%) across all state-of-the-art models, even though the added clause does not contribute to the reasoning chain needed to reach the final answer. Overall, our work provides a more nuanced understanding of LLMs’ capabilities and limitations in mathematical reasoning,” the researchers wrote.
WHAT IT MATTERS
Experts, including Microsoft’s Harjinder Sandhu, CTO of health platforms and solutions at Microsoft, sat down with HIMSS TV to discuss how the new domain of LLMs is fundamentally different from previous models and the importance of building frameworks optimized for reliability and accuracy to ensure patient safety.
As LLMs are continually being used within healthcare, many experts and researchers highlight the necessity of providers to fully understand AI’s objectives and its potential use in clinical practice. It’s also crucial to ensure proper use cases of the technology and how healthcare applications are utilizing LLMs.
A systemic review published earlier this week in JAMA Network examined how healthcare applications of LLMs were being evaluated.
Researchers found that of 519 studies published between Jan. 1, 2022, and Feb. 19. 2024, only 5% used actual patient care data to evaluate their LLMs.
Results of the review suggested that current LLM evaluation in healthcare was “fragmented and insufficient and that evaluations need to use real patient data, quantify biases, cover a wider range of tasks and specialties and report standardized performance metrics to enable broader implementation.”