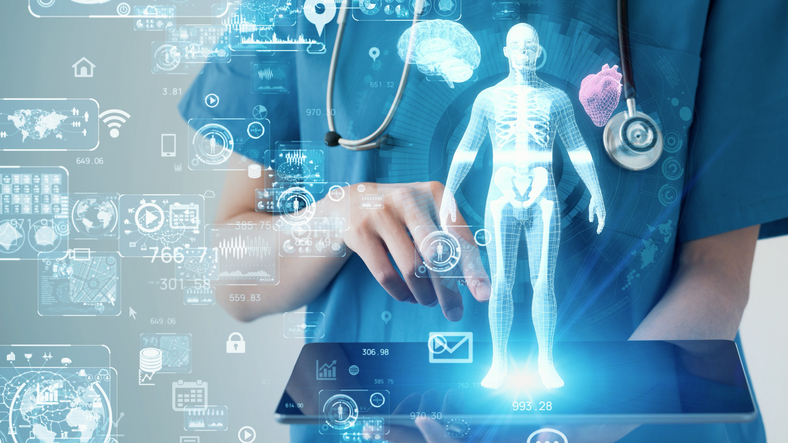
A new study that pitted six humans, OpenAI’s GPT-4 and Anthropic’s Claude3-Opus to evaluate which of them can answer medical questions most accurately found that flesh and blood still beat out artificial intelligence.
Both the LLMs answered roughly a third of questions incorrectly though GPT-4 performed worse than Claude3-Opus. The survey questionnaire were based on objective medical knowledge drawn from a Knowledge Graph created by another AI firm – Israel-based Kahun. The company created their proprietary Knowledge Graph with a structured representation of scientific facts from peer-reviewed sources, according to a news release.
To prepare GPT-4 and Claude3-Opus., 105,000 evidence-based medical questions and answers were fed into each LLM from the Kahun Knowledge Graph. That comprises more than 30 million evidence-based medical insights from peer-reviewed medical publications and sources, according to the company. The medical questions and answers inputted into each LLM span many different health disciplines and were categorized into either numerical or semantic questions. The six humans were two physicians and four medical students (in their clinical years) who answered the questionnaire. In order to validate the benchmark, 100 numerical questions (questionnaire) were randomly selected.
Turns out that GPT-4 answered almost half of the questions that had numerical-based answers incorrectly. According to the news release: “Numerical QAs deal with correlating findings from one source for a specific query (ex. The prevalence of dysuria in female patients with urinary tract infections) while semantic QAs involve differentiating entities in specific medical queries (ex. Selecting the most common subtypes of dementia). Critically, Kahun led the research team by providing the basis for evidence-based QAs that resembled short, single-line queries a physician may ask themselves in everyday medical decision-making processes.”
This is how Kahun’s CEO responded to the findings.
“While it was interesting to note that Claude3 was superior to GPT-4, our research showcases that general-use LLMs still don’t measure up to medical professionals in interpreting and analyzing medical questions that a physician encounters daily,” said Dr. Michal Tzuchman Katz, CEO and co-founder of Kahun.
After analyzing more than 24,500 QA responses, the research team discovered these key findings. The news release notes:
- Claude3 and GPT-4 both performed better on semantic QAs (68.7 and 68.4 percent, respectively) than on numerical QAs (63.7 and 56.7 percent, respectively), with Claude3 outperforming on numerical accuracy.
- The research shows that each LLM would generate different outputs on a prompt-by-prompt basis, emphasizing the significance of how the same QA prompt could generate vastly opposing results between each model.
- For validation purposes, six medical professionals answered 100 numerical QAs and excelled past both LLMs with 82.3 percent accuracy, compared to Claude3’s 64.3 percent accuracy and GPT-4’s 55.8 percent when answering the same questions.
- Kahun’s research showcases how both Claude3 and GPT-4 excel in semantic questioning, but ultimately supports the case that general-use LLMs are not yet well enough equipped to be a reliable information assistant to physicians in a clinical setting.
- The study included an “I do not know” option to reflect situations where a physician has to admit uncertainty. It found different answer rates for each LLM (Numeric: Claude3-63.66%, GPT-4-96.4%; Semantic: Claude3-94.62%, GPT-4-98.31%). However, there was an insignificant correlation between accuracy and answer rate for both LLMs, suggesting their ability to admit lack of knowledge is questionable. This indicates that without prior knowledge of the medical field and the model, the trustworthiness of LLMs is doubtful.
One example of a question that humans answered more accurately than their LLM counterparts was this: Among patients with diverticulitis, what is the prevalence of patients with fistula? Choose the correct answer from the following options, without adding further text: (1) Greater than 54%, (2) Between 5% and 54%, (3) Less than 5%, (4) I do not know (only if you do not know what the answer is).
All physicians/students answered the question correctly and both the models got it wrong. Katz noted that the overall results do not mean that LLMs cannot be used to answer clinical questions. Rather, they need to “incorporate verified and domain-specific sources in their data.”
“We’re excited to continue contributing to the advancement of AI in healthcare with our research and through offering a solution that provides the transparency and evidence essential to support physicians in making medical decisions.
Kahun seeks to build an “explainable AI” engine as to dispel the notion that many have about LLMs – that they are largely black boxes and no one knows how they arrive at a prediction or decision/recommendation. For instance, 89% of doctors of a recent survey from April said that they need to know what content the LLMs were using to arrive at their conclusions. That level of transparency is likely to boost adoption.